7. 稳定扩散模型(Stable diffusion model)¶
DDPM 模型在生成图像质量上效果已经非常好,但它也有个缺点, 那就是 \(x_t\) 的尺寸是和图片一致的,\(x_t\) 的元素和图片的像素是一一对应的, 所以称 DDPM 是像素(pixel)空间的生成模型。 我们知道一张图片的尺寸是 \(3 \times H \times W\) ,如果想生成一张高尺寸的图像, \(x_t\) 的张量大小是非常大的,这就需要极大的显卡(硬件)资源,包括计算资源和显存资源。 同样的,它的训练成本也是高昂的。高昂的成本极大的限制了它在民用领用的发展。
7.1. 潜在扩散模型(Latent diffusion model,LDM)¶
2021年德国慕尼黑路德维希-马克西米利安大学计算机视觉和学习研究小组(原海德堡大学计算机视觉小组), 简称 CompVis 小组,发布了论文 High-Resolution Image Synthesis with Latent Diffusion Models [1],针对这个问题做了一些改进, 主要的改进点有:
引入一个自编码器,先对原始对象进行压缩编码,编码后的向量再应用到扩散模型。
通过在 UNET 中加入 Attention 机制,处理条件变量 \(y\)。
潜在空间
针对 DDPM 消耗资源的问题,解决方法也简单。 引入一个自编码器,比如上一章介绍的变分编码器(VAE),先对原始图像进行压缩编码,得到图像的低维表示 \(z_0\) ,然后 \(z_0\) 作为 DDPM 的输入,执行 DDPM 的算法过程,DDPM 生成的结果再经过解码器还原成图像。 由于 \(z_0\) 是压缩过的,其尺寸远远小于原始的图像,这样就能极大的减少 DDPM 资源的消耗。 压缩后 \(z_0\) 所在的数据空间称为潜在空间(latent space), \(z_0\) 可以称为潜在数据。
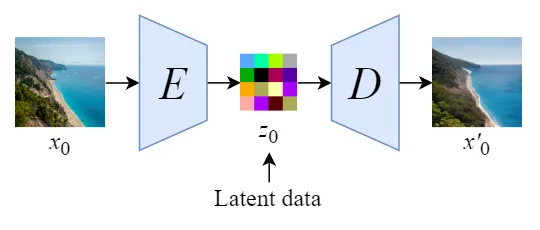
这个自编码器(VAE)可以是提前预训练好的模型,在训练扩散模型时,自编码器的参数是冻住的, 如 图 7.1.2 所示
通过使用预训练的编码器 \(E\),我们可以将全尺寸图像编码为低维潜在空间数据(压缩数据)。
通过使用预训练的解码器 \(D\),我们可以将潜在空间数据解码回图像。
这样在 DDPM 外层增加一个 VAE 后,DDPM 的扩散过程和降噪过程都是在潜空间(Latent Space)进行, 潜空间的尺寸远远小于像素空间,极大了降低了硬件资源的需求,同时也能加速整个过程。
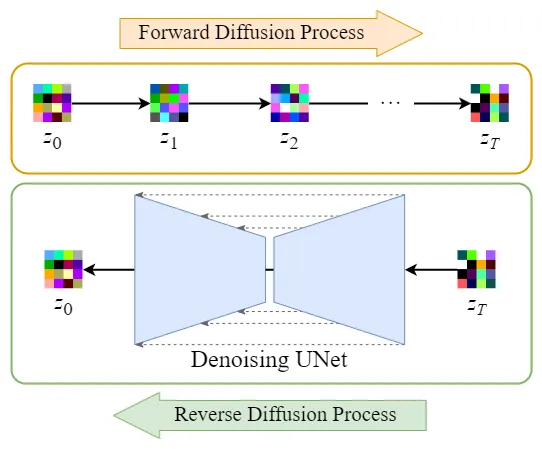
正向扩散过程→给潜在数据增加噪声,逆向扩散过程→从潜在数据中消除噪声。 整个 DDPM 的过程都是在潜在空间执行的, 所以这个算法被称为潜在扩散模型(Latent diffusion model,LDM)。 增加一个自编码器并没有改变 DDPM 的算法过程,所以并不需要对 DDPM 算法代码做任何改动。
条件处理
在 DDPM 的过程中,可以增加额外的指导信息,使其生成我们的想要的图像, 比如文本生成图像、图像生成图像等等。
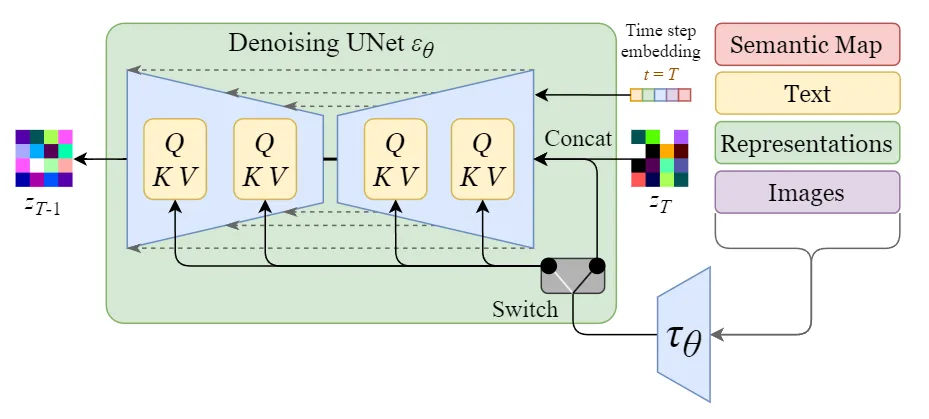
用符号 \(y\) 表示额外的条件数据,用 \(\tau_{\theta}\) 表示 \(y\) 的加工处理过程,它负责把 \(y\) 加工成特征向量。 比如,如果 \(y\) 是一段文本的 prompt, \(\tau_{\theta}\) 就是可以是一个 text-encoder, 论文中使用的预训练好的 CLIP 模型中的 text-encoder。 之所以用 CLIP 模型的 text-encoder, 是因为 CLIP 模型本身就是一个文本图像的多模态模型, 它的 text-encoder 能更贴近图像的特征空间, 这里选用一个预训练好的 CLIP 模型即可。
通过在 UNET 网络中增加 Attention 机制把文本的嵌入向量( \(\tau_{\theta}(y)\) ) 加入到 UNET 网络中。加入不同的内容可以通过一个开关(switch)来控制, 如 图 7.1.4 所示。
对于文本输入,它们首先使用语言模型 \(\tau_{\theta}(y)\) (例如BERT,CLIP)转换为嵌入(向量),然后通过(多头)注意(Q,K,V)层映射到U-Net。
对于其他空间对齐的输入(例如语义图、图像、修复),可以使用串联来完成调节。
关于注意力机制的实现细节,可以直接参考论文代码, LDM模型论文的代码和预训练的模型已经在 Github 开源,地址为: https://github.com/CompVis/latent-diffusion 。
训练过程
相比于 DDPM ,条件化的 LDM 目标函数稍微变化了一点,具体变化内容可以参考 图 7.1.5。
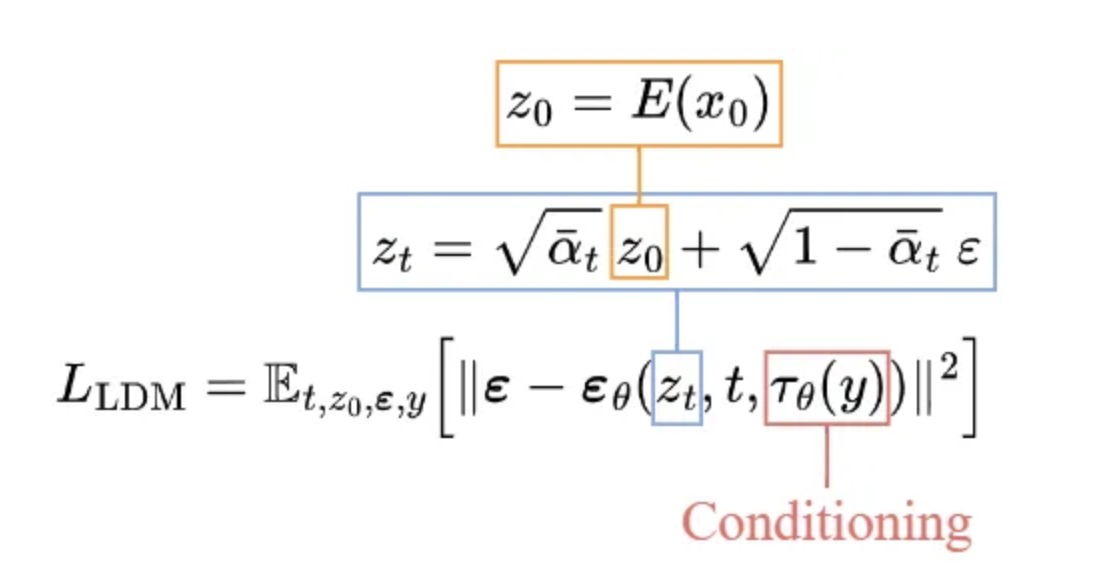
生成(采样)过程
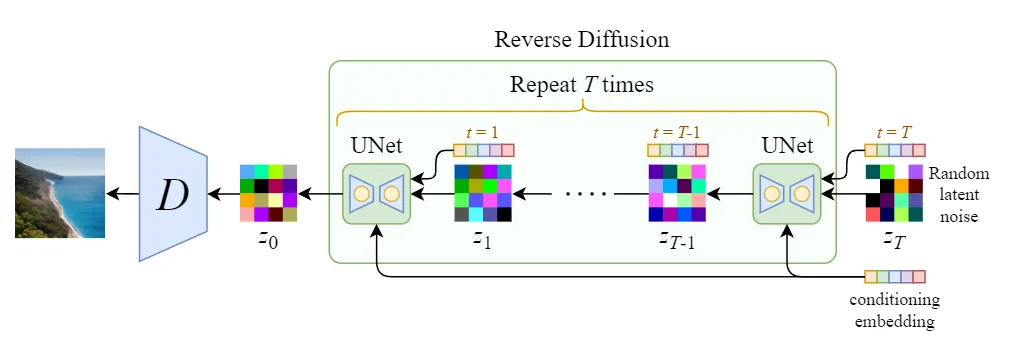
图 7.1.6 是 LDM 采样过程的图形化表示, 过程并不复杂,经过 DDPM 采样生成的 \(z_0\) 需要用解码器 \(D\) 还原成图像。
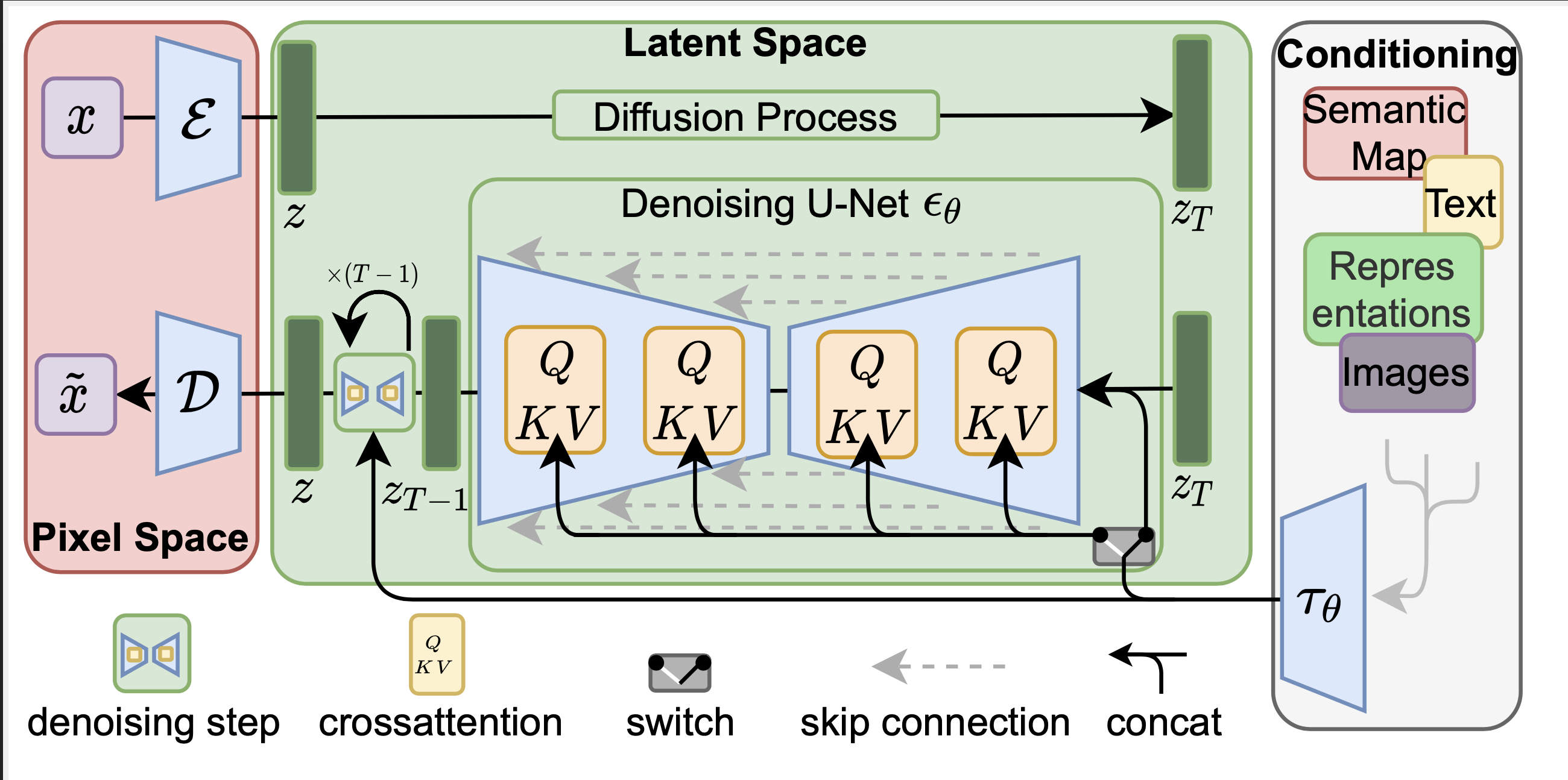
7.2. 稳定扩散模型(Stable diffusion,SD)¶
LDM 本身是由 CompVis 提出并联合 Runway ML进行开发实现,后来 Stability AI 也参与进来并提供了一些资源, 联合搞了一个预训练的 LDM 模型,称为 Stable diffusion。 所以,Stable diffusion 是 LDM 的一个开源预训练模型,由于它的开源迅速火爆起来。 目前 Stable diffusion 已经占据了图像生成开源领域的主导地位。
由于 Stable diffusion 只是LDM的一个开源预训练模型,没有额外的复杂数学公式需要讨论, 这里我们就直接上代码吧。 我们不用 Stable diffusion 的官方代码库 stablediffusion ,而是 huggingface 开源库 diffusers 中的实现, 它的易读性更好一些。
7.2.1. 推理过程代码¶
diffusers 把模型的核心逻辑都封装在各种 DiffusionPipeline 中,
StableDiffusionPipeline 核心代码在 diffusers.StableDiffusionPipeline
先看初始化代码,可明显看到整个 StableDiffusionPipeline 包含几个关键组件:vae,text_encoder/tokenizer,unet,scheduler。
这几个组件和 LDM 中是对应的。
vae: VAE 自编码器,负责前后的编解码(压缩、解压缩)工作。
text_encoder/tokenizer: 文本编码器,负责对文本Prompt进行编码处理。
unet: 噪声预测模型,也是DDPM的核心。
scheduler: 负责降噪过程(逆过程)的计算,也就是实现 \(x_t -> x_{t-1}\) ,对应着 DDPM、DDIM、ODE等不同的降采样实现。
safety_checker: 做生成图像安全性检查的,可选,暂时可以不关注它。
feature_extractor: 如果输入条件中存在 img,也就是以图生图(img2img),可以用它对条件图片进行特征抽取,也就是图像编码器(img encoder),可选。
def __init__(
self,
vae: AutoencoderKL,
text_encoder: CLIPTextModel,
tokenizer: CLIPTokenizer,
unet: UNet2DConditionModel,
scheduler: KarrasDiffusionSchedulers,
safety_checker: StableDiffusionSafetyChecker,
feature_extractor: CLIPImageProcessor,
requires_safety_checker: bool = True,
):
...
接下来是核心逻辑的实现,代码在方法 StableDiffusionPipeline::__call__
中,
我们直接在代码加注释予以解说。
def __call__(
self,
prompt: Union[str, List[str]] = None,
height: Optional[int] = None,
width: Optional[int] = None,
num_inference_steps: int = 50,
guidance_scale: float = 7.5,
negative_prompt: Optional[Union[str, List[str]]] = None,
num_images_per_prompt: Optional[int] = 1,
eta: float = 0.0,
generator: Optional[Union[torch.Generator, List[torch.Generator]]] = None,
latents: Optional[torch.FloatTensor] = None,
prompt_embeds: Optional[torch.FloatTensor] = None,
negative_prompt_embeds: Optional[torch.FloatTensor] = None,
output_type: Optional[str] = "pil",
return_dict: bool = True,
callback: Optional[Callable[[int, int, torch.FloatTensor], None]] = None,
callback_steps: int = 1,
cross_attention_kwargs: Optional[Dict[str, Any]] = None,
):
r"""
Function invoked when calling the pipeline for generation.
Args:
prompt (`str` or `List[str]`, *optional*):
The prompt or prompts to guide the image generation. If not defined, one has to pass `prompt_embeds`.
instead.
height (`int`, *optional*, defaults to self.unet.config.sample_size * self.vae_scale_factor):
The height in pixels of the generated image.
width (`int`, *optional*, defaults to self.unet.config.sample_size * self.vae_scale_factor):
The width in pixels of the generated image.
num_inference_steps (`int`, *optional*, defaults to 50):
The number of denoising steps. More denoising steps usually lead to a higher quality image at the
expense of slower inference.
guidance_scale (`float`, *optional*, defaults to 7.5):
Guidance scale as defined in [Classifier-Free Diffusion Guidance](https://arxiv.org/abs/2207.12598).
`guidance_scale` is defined as `w` of equation 2. of [Imagen
Paper](https://arxiv.org/pdf/2205.11487.pdf). Guidance scale is enabled by setting `guidance_scale >
1`. Higher guidance scale encourages to generate images that are closely linked to the text `prompt`,
usually at the expense of lower image quality.
negative_prompt (`str` or `List[str]`, *optional*):
The prompt or prompts not to guide the image generation. If not defined, one has to pass
`negative_prompt_embeds` instead. Ignored when not using guidance (i.e., ignored if `guidance_scale` is
less than `1`).
num_images_per_prompt (`int`, *optional*, defaults to 1):
The number of images to generate per prompt.
eta (`float`, *optional*, defaults to 0.0):
Corresponds to parameter eta (η) in the DDIM paper: https://arxiv.org/abs/2010.02502. Only applies to
[`schedulers.DDIMScheduler`], will be ignored for others.
generator (`torch.Generator` or `List[torch.Generator]`, *optional*):
One or a list of [torch generator(s)](https://pytorch.org/docs/stable/generated/torch.Generator.html)
to make generation deterministic.
latents (`torch.FloatTensor`, *optional*):
Pre-generated noisy latents, sampled from a Gaussian distribution, to be used as inputs for image
generation. Can be used to tweak the same generation with different prompts. If not provided, a latents
tensor will ge generated by sampling using the supplied random `generator`.
prompt_embeds (`torch.FloatTensor`, *optional*):
Pre-generated text embeddings. Can be used to easily tweak text inputs, *e.g.* prompt weighting. If not
provided, text embeddings will be generated from `prompt` input argument.
negative_prompt_embeds (`torch.FloatTensor`, *optional*):
Pre-generated negative text embeddings. Can be used to easily tweak text inputs, *e.g.* prompt
weighting. If not provided, negative_prompt_embeds will be generated from `negative_prompt` input
argument.
output_type (`str`, *optional*, defaults to `"pil"`):
The output format of the generate image. Choose between
[PIL](https://pillow.readthedocs.io/en/stable/): `PIL.Image.Image` or `np.array`.
return_dict (`bool`, *optional*, defaults to `True`):
Whether or not to return a [`~pipelines.stable_diffusion.StableDiffusionPipelineOutput`] instead of a
plain tuple.
callback (`Callable`, *optional*):
A function that will be called every `callback_steps` steps during inference. The function will be
called with the following arguments: `callback(step: int, timestep: int, latents: torch.FloatTensor)`.
callback_steps (`int`, *optional*, defaults to 1):
The frequency at which the `callback` function will be called. If not specified, the callback will be
called at every step.
cross_attention_kwargs (`dict`, *optional*):
A kwargs dictionary that if specified is passed along to the `AttentionProcessor` as defined under
`self.processor` in
[diffusers.cross_attention](https://github.com/huggingface/diffusers/blob/main/src/diffusers/models/cross_attention.py).
Examples:
Returns:
[`~pipelines.stable_diffusion.StableDiffusionPipelineOutput`] or `tuple`:
[`~pipelines.stable_diffusion.StableDiffusionPipelineOutput`] if `return_dict` is True, otherwise a `tuple.
When returning a tuple, the first element is a list with the generated images, and the second element is a
list of `bool`s denoting whether the corresponding generated image likely represents "not-safe-for-work"
(nsfw) content, according to the `safety_checker`.
"""
# 0. Default height and width to unet
# unet 网络输入的图像尺寸,也就是潜空间 Latent space 的尺寸
height = height or self.unet.config.sample_size * self.vae_scale_factor
width = width or self.unet.config.sample_size * self.vae_scale_factor
# 1. Check inputs. Raise error if not correct
# 检查输入的合法性,可以不关注
self.check_inputs(
prompt, height, width, callback_steps, negative_prompt, prompt_embeds, negative_prompt_embeds
)
# 2. Define call parameters
if prompt is not None and isinstance(prompt, str):
batch_size = 1
elif prompt is not None and isinstance(prompt, list):
batch_size = len(prompt)
else:
batch_size = prompt_embeds.shape[0]
device = self._execution_device
# here `guidance_scale` is defined analog to the guidance weight `w` of equation (2)
# of the Imagen paper: https://arxiv.org/pdf/2205.11487.pdf . `guidance_scale = 1`
# corresponds to doing no classifier free guidance.
# 是否启动 classifier_free_guidance 特性,如果启用的需要同时执行带条件的噪声预测和不带条件的噪声预测
# 注意,负提示词是否生效和它相关,只有启用 classifier_free_guidance 负提示词才会生效,
# 否则负提示词不起作用。
do_classifier_free_guidance = guidance_scale > 1.0
# 3. Encode input prompt
# 对输入的文本Prompt进行编码处理,内部其实是调用文本编码器进行编码处理
# 这里需要注意的是负提示词的处理
# 如果 do_classifier_free_guidance == True,负提示词才会生效,并起是和正提示词在batch维度拼接在一起
# prompt_embeds = torch.cat([negative_prompt_embeds, prompt_embeds])
# 稍后解释为什么
prompt_embeds = self._encode_prompt(
prompt,
device,
num_images_per_prompt,
do_classifier_free_guidance,
negative_prompt,
prompt_embeds=prompt_embeds,
negative_prompt_embeds=negative_prompt_embeds,
)
# 4. Prepare timesteps
self.scheduler.set_timesteps(num_inference_steps, device=device)
timesteps = self.scheduler.timesteps
# 5. Prepare latent variables
# unet 网络输入支持的 channels 数量
num_channels_latents = self.unet.config.in_channels
# 设置降噪过程的初始化随机高斯噪声,也就是 latent 初始化
latents = self.prepare_latents(
batch_size * num_images_per_prompt,
num_channels_latents,
height,
width,
prompt_embeds.dtype,
device,
generator,
latents,
)
# 6. Prepare extra step kwargs. TODO: Logic should ideally just be moved out of the pipeline
extra_step_kwargs = self.prepare_extra_step_kwargs(generator, eta)
# 7. Denoising loop
num_warmup_steps = len(timesteps) - num_inference_steps * self.scheduler.order
with self.progress_bar(total=num_inference_steps) as progress_bar:
for i, t in enumerate(timesteps):
# expand the latents if we are doing classifier free guidance
# 这里在 batch 维度放大两倍,是为了在一个batch中同时处理得到有条件噪声预测和无条件噪声预测
latent_model_input = torch.cat([latents] * 2) if do_classifier_free_guidance else latents
latent_model_input = self.scheduler.scale_model_input(latent_model_input, t)
# predict the noise residual
# 预测噪声,当然这个过程是在潜空间 latent space 进行的
noise_pred = self.unet(
latent_model_input,
t,
encoder_hidden_states=prompt_embeds,
cross_attention_kwargs=cross_attention_kwargs,
return_dict=False,
)[0]
# perform guidance
if do_classifier_free_guidance:
# 在batch维度一分为二,前一半作为无条件预测噪声,后一边是有条件预测噪声
noise_pred_uncond, noise_pred_text = noise_pred.chunk(2)
# 根据 classifier free guidance 公式进行加权求和
noise_pred = noise_pred_uncond + guidance_scale * (noise_pred_text - noise_pred_uncond)
# compute the previous noisy sample x_t -> x_t-1
# 使用 scheduler 进行降噪处理,这里 scheduler 其实就是不同降噪采样算法的实现,可以有多种不同实现,比如 DDPM、DDIM、ODE等各种采样算法
latents = self.scheduler.step(noise_pred, t, latents, **extra_step_kwargs, return_dict=False)[0]
# call the callback, if provided
if i == len(timesteps) - 1 or ((i + 1) > num_warmup_steps and (i + 1) % self.scheduler.order == 0):
progress_bar.update()
if callback is not None and i % callback_steps == 0:
callback(i, t, latents)
# 最后,还需要用 vae 的解码器,把 latent 解码成原尺寸的图像
if output_type == "latent":
image = latents
has_nsfw_concept = None
elif output_type == "pil":
# 8. Post-processing
image = self.decode_latents(latents)
# 9. Run safety checker
image, has_nsfw_concept = self.run_safety_checker(image, device, prompt_embeds.dtype)
# 10. Convert to PIL
image = self.numpy_to_pil(image)
else:
# 8. Post-processing
image = self.decode_latents(latents)
# 9. Run safety checker
image, has_nsfw_concept = self.run_safety_checker(image, device, prompt_embeds.dtype)
# Offload last model to CPU
if hasattr(self, "final_offload_hook") and self.final_offload_hook is not None:
self.final_offload_hook.offload()
if not return_dict:
return (image, has_nsfw_concept)
return StableDiffusionPipelineOutput(images=image, nsfw_content_detected=has_nsfw_concept)
整个实现是非常清晰简洁的,很容易能看懂,这里稍微复杂的地方就是 classifier_free_guidance 的处理, 先回顾一下 classifier_free_guidance 核心的公式
前面讨论过 classifier free guidance 技术,其实实现起来很简单,就是降噪过程中,用同一个 UNET 网络分别进行有条件和无条件两个噪声预测, 然后两者加权求和作为最终的预测噪声。 这里实现的时候有两个小 trick:
没有分别调用 UNET 两次,而是把输入 batch 扩大两倍,前面部分作为无条件,后面部分作为有条件,反正都是同一个 UNET 网络,这样做效率更高。
无条件部分,并不是真的没有任何条件,而是把负提示词作为条件,所以是把负提示词和 classifier_free_guidance 糅合在一起实现了。
另外一点,用 VAE 编码成的latent,其实可以看做是一个更小尺寸的压缩图片,比如目标图像是 \(256 \times 256\) ,经过用 VAE 编码(压缩后)后变成 \(64 \times 64\) 的小尺寸图像, 最后再用 VAE 的解码器放大到 \(256 \times 256\)。 所以其实,VAE 可以看做一个图片压缩解压缩的过程,那我们是不是可以利用它做图像的高清修复呢。
7.2.2. 训练过程¶
训练过程的代码在 diffusers/examples/text_to_image/ 目录下,目前有三个版本。
待补充